- Financial data simplified
Financial data simplified
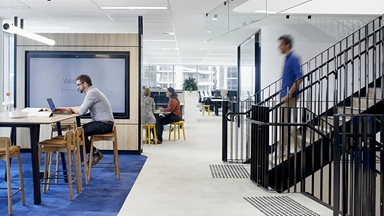
Content Summary
Podcast episode
Speaker 1:
Hello and welcome to the CPA Australia Podcast. Your weekly source for accounting, education, career and leadership discussion.Jana Schmitz:
Hello and welcome to CPA Australia's ACT Public Sector Committees Podcast. I am Dr. Jana Schmitz, CPA Australia's Digital Economy policy lead. This podcast is being recorded on Friday the 9th of September 2022. In this podcast episode today, we will discuss different aspects of financial data and how financial data can be combined with other data to provide meaningful information and business insights. The field and scope of financial data and data more generally is so broad that one single podcast episode would not do it justice. So, today we are going to focus on selected key areas that affect CPA Australia members and the accounting profession more broadly. And these key areas are data triangulation, data governance, and data sovereignty. To help us delve into these three key areas, it's my pleasure to introduce Doug Boyd, Director at Data ReFactory. Doug is a tech evangelist who has spent more than 20 years in technology leadership roles. Welcome back and thank you for joining us today.Douglas Boyd:
Thank you very much Jana. It's a pleasure to be here and I'd like to just thank the CPA for inviting me. I'd also like to say that it's quite daunting to see that I have more than 20 years in the industry, [inaudible 00:01:42].Jana Schmitz:
Time flies.Douglas Boyd:
Oldest data we're going to talk about.Jana Schmitz:
Exactly. Yeah. So Doug, can you lead us off by taking us back to the basics and tell us what data is?Douglas Boyd:
Oh man. Okay. That's a really broad front, but let's see if we can box it in a bit. Data is any piece of information at the fine grain layer. It's information which is collected from an action or from a system or from an observation. While we like to think of data as related to a specific function, I.e. Financial, in the case of the audience here, it's really helpful to break that paradigm and think of data is simply nothing more than data. It's a small piece of zeros and ones, and it may relate to other pieces or it may be standalone. Now, taking it a step further, we can try to define financial data as any data which can be used in a financial setting. However, this breaks and you can't think of financial data because the same data may be useful by other parts of the business of the enterprise. So, data may be financial because it's used by the financial sector, or it may be operational, same piece of data because it's used by somebody in an operational sense. So rather than get into the hole, this is financial, that's operational, and over here's a different type of data. I prefer to think of data as an agnostic thing. It is a small grain piece of zeros or ones, and it is simply useful to whoever may find it useful. The last thing I'll say about data is how actually useless it is. And this is a pretty important thing because people say, "Oh, data it's so useful." Data is not useful. Data is useless. It is expensive, it's painful. Data is so granular as to tell specifics about a single event that in a macro setting it doesn't tell you anything. Let's take for instance, a single piece of data from a census might be something like the amount of income for a single family located at a specific address, 84 at Adelphi Street, Rouse Hill, new South Wales, for instance. Maybe they have an income of $98,000. Is that piece of information useful? No, it has no value to us. But if we take 80,000 pieces of the same type of data, income per household, we aggregate it up and you get an idea of a demographic of a suburb. Now that is actually somewhat useful, but it still doesn't tell much of a story. That moves from data into information. Information still isn't all that useful either from a macro sense, but if you get enough information, you can aggregate or curate it into business intelligence, and that's where we get usefulness. So data, useless. Information, relatively useless. Business intelligence, very, very useful. Does that make sense?Jana Schmitz:
Absolutely, Doug. And that brings me to the data triangulation topic. So, what exactly is data triangulation, and how is it used? So, how does it work?Douglas Boyd:
Okay, so bear with me on this. I'll try to keep it. I'm going to take us a little bit outside of the data and financial area, but work with me on it. So, data triangulation is when you take seemingly unrelated data sets and find your way to relate them together. Through that, you're going to reveal or into it a new data set, which is unrelated to the first couple of data sets you're working with. Think of it as alchemy of data where you're taking disparate pieces and you're combining them to make gold. A couple of simple examples will help explain this. Here at Data ReFactory, we've got some work going on with the Department of Defence. We're working with their ERP project on roll migration for their migration to the SAP platform. This work involves taking position data, I.e. Cook, mechanic, deckhand, pilot, ships captain, whatever, and mapping it from the current position, what they call it today into the new world of ERP. So, a cook may for instance today be called a cook, and in the new ERP world, it may be called a chef's hand, or it may be called a salad maker, or it may be called a cook. It could be a one for one map, it changes. So, the data file that we work with contains physical location and position. It does not contain personnel data because the Department of Defence consider this unsecure if we use personnel data. Work with me on this. If we take a look at that data, we can map cook's hand to cook or ships' captain to captain of vessel, whatever they call it. But if we dig into it a bit, we find that that simple pattern reveals a lot more than is just there. So, we take a look at a single day, a single snapshot doesn't tell us much, but over time we can see movements of positions around the country and around the world. From that, we can triangulate new meaning. For instance, if I walk through the data every two-week intervals and aggregate position counts against a location, in early February I note that in Garden Island in Sydney, there were 40 cooks. There were 32 aircraft mechanics and there were 10 ships captains. In late February, Garden Island shrank to only 15 cook positions while the aircraft mechanics stayed the same and the ships' captain stayed the same. Then in March, the cook positions jump back up. If you're just looking at one slice, you won't get it. But if you compare those three slices together, you can figure out, without too much digging around that there was a major training exercise that happened in far north Queensland and that it was not a deployment of everybody because they only took up the pilots and the aircraft and a couple of cooks to feed them. They didn't actually have to move the mechanics to fix the planes because they weren't going to be up there that long. We've taken a single data set, we've looked at it across time, and we've figured out major motion of personnel. Does that make sense?Jana Schmitz:
Absolutely. And what I'm hearing Doug, is that data triangulation has a lot of benefits, but also there might be some disadvantages. So, could you touch on the benefits of data triangulation from a strategic and a decision-making perspective, and perhaps also give us some insights into the potential disadvantages of data triangulation?Douglas Boyd:
Cool. Okay. So, as we've looked at already, the business benefits are potentially huge but they do come with a cost, both financial and with a risk cost as well. Using triangulation techniques as an example, allows us to interrelate weather forecasting data with economic data, that's really perfect for forecasting insurance costs on specific geophysical location activity, and really it's limited only by your imagination is how to relate seemingly unrelated data sets. Your imagination is the limit in making stuff fit together here. The cost on a personnel point of view is relatively simple as well. All it takes is time and two specific skill sets to put it together. The first, as I touched on, is the imaginative mind to try and relate the unrelatable aspects of the data. Election cycles to household incomes, for instance, I haven't dug into that one personally but I can see that that would be a relatively fun thing to play around with. We want to track household incomes, we want to look at the election cycles, and then we want to throw across the top of it, for instance, local elections and see if there's any sort of interrelated patterns that we can track down. Suburb location to car purchase, now that would be a fun one to play with as well. The skill set I'm trying to get here is not one of data and analytics, but one of imagination. Trying to figure out the big picture. Don't look at the numbers, look at the society, look at whatever it is you're trying to track. Try to get the idea of it. Trying to find a way to predetermine if a certain type of business will succeed or fail in a specific area, that's a classic one. You want to see risk, you want to understand insurance costs, you want to understand any number of elements. Try to look at all of the pieces from a holistic view of how that data fits together. That's skill number one. The second skill set is the analytic ability to be able to gather all of the data and perform the complex analysis using modern tools. It's one thing to have a hunch and say, "Okay, every time that labour comes in, prices go up or prices go down, or prices stay the same." That thought going into it is a false positive and a bias. You need to have the skills to be able to provide analysis across the data that you're working with, so that you do not reaffirm biases and predetermined thought before you started the exercise. That's a skill that takes time, takes years to work with. It also takes good training. Personally, I believe data analysts are born not particularly trained. You have to have a certain mindset which an individual has to have. Now, once they've got that mindset and they think scientifically that would be the correct way to say it, then you can certainly train them up. But if somebody doesn't have the ability to think critically, then they're really not going to be able to approach data and analytics in a way that's going to give meaningful data. The second type of cost that I'll touch on is risk. When you release data out there, you don't know what clever person is going to be looking at it, triangulating around you. Facebook, classic case in point, you look at the data that they release and the data that they capture from us. There have been entire industries built on predictive analysis of what is likely to make you click on a link and what is likely make you stay on a page and commit your hard earned dollars or influence the way you think. Cambridge Analytics, are you familiar with that Jana? Do you know the name?Jana Schmitz:
Yeah, absolutely. Yeah.Douglas Boyd:
So, that's a classic case of we are going to analyse a small subset of the population who are influenceable to try to influence their vote because we only need, what was it, 2.53% of the vote to shift, and you've got a changed election outcome. All of that was done in the States and it's now publicly available knowledge. You can see how they did it. If you can do that with predictive analysis all through data triangulation, which is publicly available data, you begin to see some of the risk. Going back to the Department of Defence information that they declare that information to be open and not sensitive. As soon as we saw its potential value of triangulation within that data set, we refuse to take it on site here. It must be stored on Department of Defence servers, so it doesn't go out into the wild under our watch. Just as an aside there on risk mitigation and the risks of data triangulation.Jana Schmitz:
That was such a comprehensive response. I'm still thinking about data, sorry, Cambridge Analytics, which was probably one of the most unethical data management, yeah issues. I want to touch on data governance Doug, what exactly do we mean when we talk about data governance, and why has it become so important?Douglas Boyd:
Let's start with the word governance. Okay. What is governance? Governance of the rules of the game, which we have all agreed to play ... let's go [with] swimming. The rules for swimming are very straightforward. You perform the appropriate stroke, whether it's breaststroke, back backstroke, butterfly, fly, or a regular fly. You stay in your lane and the first person to touch the wall at the end wins. It's about as straightforward as you get. There's no fighting, there's no hitting people over the head with a pool noodle. There's no scrums. You can't scream at your opponent because you're underwater. If somebody doesn't abide by the rules, by the governance of the game, they're disqualified. Governance is the rules that you agree to abide by before you stand on the blocks and agree to swim, that's governance. Data governance is the same thing. It is a simple set of rules which are adhered to by team members who deal with data. I'll say it again. Rules agreed to, adhered to by team members who deal with data. Data governance applies to the people, not to the data. People get governance messed up all the time. They say, "Well, we got data. It's under governance." Sorry, data doesn't understand governance any more than pool water understands rules for swimming, right? Sure there's governance people in a pool who handle the governance of the water, they make sure that it's heated to the correct level and that it's safe to swim in, but the water is just that. It's water. No, it doesn't care. The data doesn't care if governance is applied to or not. Now with data governance, you have a set of rules which is created by somebody over the top of it or a group or a governance committee. Ultimately, though it is a clearly defined or should be a clearly defined documented rules of engagement of how to deal with data. For instance, when a new set of data comes into organisation X, it is reviewed by person Y. It's sanitised by process M, and then it's loaded into data storage. Element Z. Pretty straightforward. One thing to remember, the rules don't actually matter. Any rule provided it is agreed to by those who are enforcing, it will work. The trick here is to make sure that the rules are agreed to by all who were enforcing it. Does that make sense?Jana Schmitz:
Yeah. And now I'm wondering where exactly does the accountants fit in there in the data governance? What are the roles that the accountants play?Douglas Boyd:
And this is the joy of governance, right? The accountant has no play here. The individual has the play here. Individual acting as a team has a play here. If they happen to be accountants, great. If they don't, again, great. It depends upon the governance structure, the rules that are around it. Now, if an accountant is working solo, say in their own business, they may have rules of governance that apply towards document storage. There's a nice easy one, which is we're going to practise safe document hygiene. Every time somebody takes a document out of the Compactus, they're going to record it upon the register. They're going to do whatever they have to do. They're going to update the notes on the front of the file. They're going to record their time, and then they're going to put it back in the Compactus. Very simple rule. If people choose not to follow the rules of governance, then documents end up all over the place. Time sheets aren't updated, and when Mr. Smith comes in, you can't find what he's been up to. Governance doesn't have to be complex. It shouldn't actually be complex. I'm going to go off topic a little bit here and discuss something called expressway governance. It's kind of pet of mine. So, often you go into places and you find that people have governance structured for the sake of governance. They're putting rules in place just to make people jump through hoops. There is no business benefit to follow them. There's no risk mitigation for following the rules. There's no reason for the rule to exist, except for somebody way back 20, 30, 40 years ago said, no, we got to do it this way, and we've always done it this way. Expressway governance is where you look at a process and you say, "Okay, it makes sense for us to do the following framework." Using the same conversation we used a second ago with taking a file out of Compactus, updating it accordingly, updating the notes, update the time sheet, put it back in the Compactus. That's what you would do normally, so why not just put a piece of governance around it so that we have a documented structure that we can all agree this is what should be happening.Jana Schmitz:
Okay, so question. What I'm hearing is that good data governance can potentially increase the quality of data. Is that correct?Douglas Boyd:
Let's talk about quality of data. Can I ask you what you mean by quality of data, or do you want me to take a guess at it?Jana Schmitz:
I think that's the one million question, Doug.Douglas Boyd:
So, let's take data that comes in from 20 different sources. Hey, let's simplify it down. It comes in from two different sources. You get a phone book and then you get Google. Those are the two simple sources. In the phone book, you look up the phone record for Doug Boyd and you get an address and a phone number, which is presented in a one line format. In Google, you track down Doug Boyd and you see that is a Facebook profile and a LinkedIn profile, and there's a webpage and there's a directorship and blah, blah, blah, blah, blah. How do you marry the two up to get a clear picture of what Doug Boyd is? You create a model of a user, and then you put Doug Boyd's information into it. Data quality is nothing more than making sure that the data complies to a predetermined format. If you say, "Okay, all of our dates are going to be day day slash MM slash YYYY and yet somebody writes in December 15th, 2004. Okay, you still know what the date is, but that's not clean. Data quality can be that simple. So, you have a data cleansing, that's another word you're going to hear here. Data cleansing exercise, that's where you comply and get all of the data into the same format. Data quality can also apply to, is the quality of the data verifiable? Does it have a high truthful count? Let's take data quality, go back to data governance. Data governance will simply provide a set of rules that will increase your data quality because it provides a set of rules around it. How do we harvest data? How do we bring data in? Where does our data come from? Here are the clearly defined rules of engagement of what to do with the data or when to ignore it. Important safety tip on that one. Now you're going to revisit your data governance rules on a regular basis if you're an active organisation, because every time you bring data in, every time you apply some sort of transformation or some sort of business intelligence, deep dive into figuring out what your data is. Through that data, curate up into information, curate up into business intelligence. You look down in your data because your business intelligence isn't telling you anything meaningful, and you find oh, well, we've got dates backwards here. Okay, you go back and you revisit your rules of conduct and contact on data quality and how to bring it in. It's a data governance piece. So yes, data governance will most certainly, if done correctly, increase your data quality.Jana Schmitz:
Two other topics that have gained importance are data sovereignty and data security. So, these are two key priority areas that also the government is currently focusing on. Now, Doug, why should we care so much about data sovereignty and security?Douglas Boyd:
Data sovereignty in a nutshell, who is owning the physical land that your data is sitting on? When you record a file to a cloud provider, it is replicated automatically into numerous places around the world. You can determine that they're only replicated here in Australia, but there's really no guarantee that AWS is going to only hold the data here in Australia unless you have a specific contract with the cloud provider. Why this is important? Because the physical land that holds the data, the physical land that holds the building that the data is in is ultimately under the jurisdiction of whatever government is in power. So, if you want your data to never fall into the hands of the Chinese or the Brazilians or the Germans or any other country, but the data is sitting on their physical land, data is not like an embassy. Data is just like any other building and it's just sitting there. Does that explain data sovereignty a little bit.Jana Schmitz:
Yeah, interesting. You did, you did Doug. Thank you so much. And I guess I tried to summarise it in my own words. So, data sovereignty is about storage of data where it is located and stored and also-Douglas Boyd:
Physically held, yes.Jana Schmitz:
Exactly. And also who has access or who is authorised to access the data? Is that correct?Douglas Boyd:
Authorised to access the data is not quite correct because you own the data and AWS own the servers. But the physical land that it's on, if that land ever comes under question or a hostile force takes it over, or somebody breaks in and just tries to steal a server if they can identify what a server looks like in a cloud instance, then yeah, that's the only instance where you'd be concerned.Jana Schmitz:
I think we have to record another episode on that particular topic. There's a lot to unpack.Douglas Boyd:
There is.Jana Schmitz:
Absolutely. But I guess this is how we have time for today, so thank you so much for sharing your time and expertise with us Doug. It's been a great pleasure and very informative having this conversation with you today. And for our listeners, if you've got a question about any of the topics we've discussed today, any of CPS Australia's policy and advocacy work, or you'd simply like to suggest the topic for another podcast, please email [email protected]. Thank you very much for listening.Speaker 1:
Thanks for listening to the CPA Australia Podcast. For more information on today's episode, please visit the show notes at www.cpaaustralia.com.au/podcast. Never miss an episode by subscribing to our podcast on Apple Podcasts, Spotify, or Stitcher.
About this episode
In this episode, tech evangelist Doug Boyd from Data reFactory delves into different aspects of financial data.
He simplifies what it is and how financial data can be combined with other data to provide meaningful information and business insights.
Specifically, he offers his insights into key areas that relate to CPA Australia members and, more broadly, the accounting profession, such as data triangulation, data governance and data sovereignty.
Listen now.
Host: Dr Jana Schmitz, Digital Economy Policy Lead, CPA Australia
Guest: Doug Boyd, Director, Data reFactory
Subscribe to With Interest
Follow With Interest on your favourite player and listen to the latest podcast episodes